How to quantify Data Quality?
Data quality refers to data conditions based on accuracy, completeness, consistency, timeliness, and reliability.
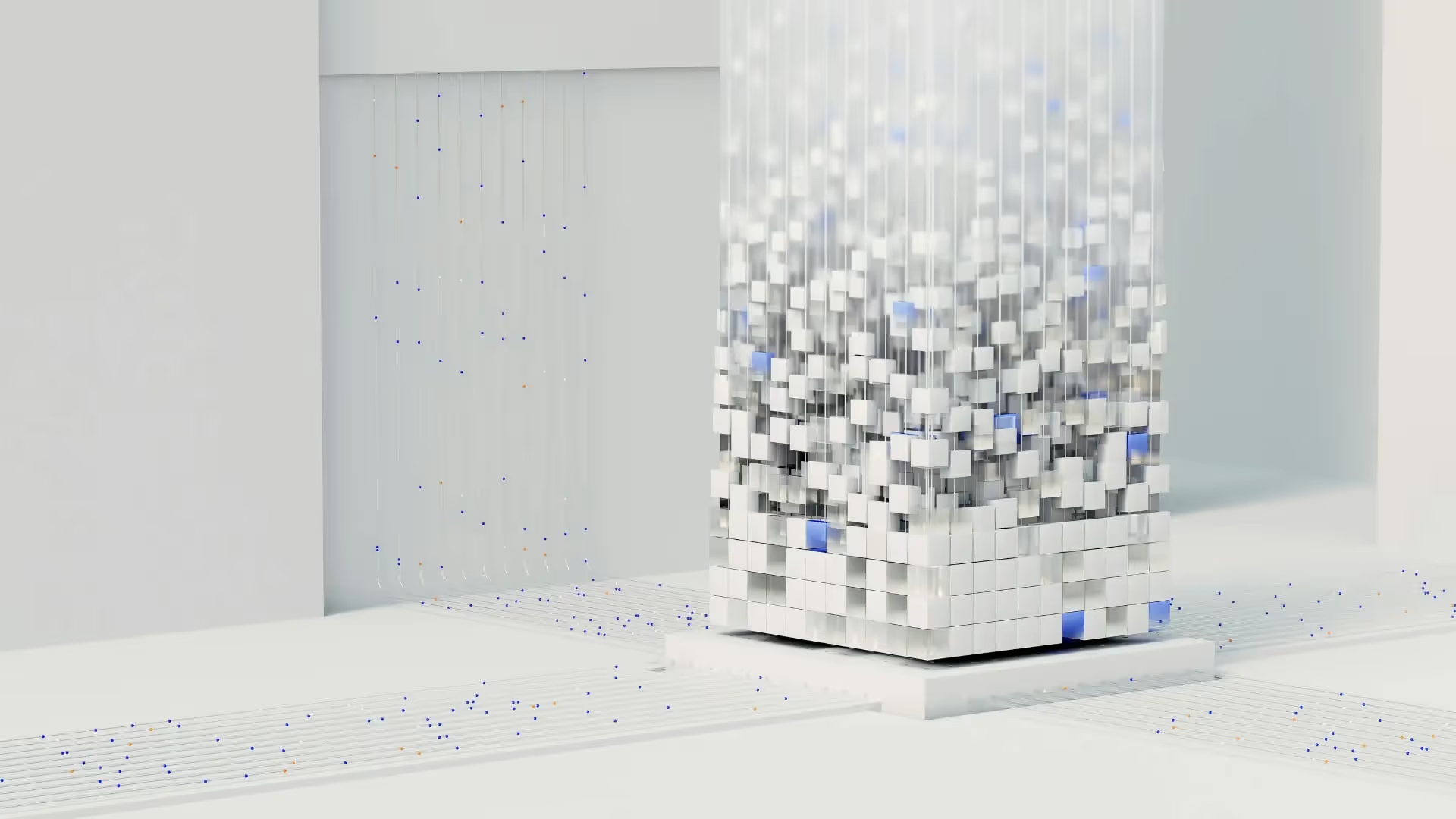
Photo by Google DeepMind on Unsplash
Introduction
In an era where data drives decisions, the quality of that data becomes paramount. Data quality is not just about having clean data; it's about ensuring it is accurate, complete, consistent, and timely. However, quantifying data quality can seem like a daunting task. With the right approach and metrics, organizations can measure and improve the quality of their data, ensuring that decisions are based on reliable information. This post delves into the nuances of quantifying data quality, offering actionable insights and strategies.
Understanding Data Quality
Data quality refers to data conditions based on accuracy, completeness, consistency, timeliness, and reliability. High-quality data must be:
- Accurate: Free from errors and inaccuracies.
- Complete: All values and data segments are present.
- Consistent: Uniform across all data sources.
- Timely: Available when needed and relevant to the current context.
- Reliable: Trustworthy and derived from credible sources.
Quantifying these dimensions allows organizations to assess the usability and reliability of their data for making decisions, setting strategies, and improving operations.
Metrics for Measuring Data Quality
Accuracy
Data accuracy is paramount; it refers to how closely data reflects the real-world values it is supposed to represent. Accuracy can be quantified by calculating the error rate, which involves comparing data entries against a verified source and determining the percentage of correct records.
Completeness
Completeness measures whether all required data is present. This can be quantified by identifying missing values or records and calculating the percentage of complete data sets.
Consistency
Consistency ensures that data across different sources or databases remains uniform and contradictory-free. It is crucial for maintaining data integrity in analysis and decision-making. Organizations can quantify consistency by measuring the number of inconsistencies found when comparing similar data from different sources, expressed as a percentage or a rate.
Timeliness
Timeliness measures how current and up-to-date the data is. In rapidly changing environments, the value of data can diminish over time, making timeliness a critical quality dimension. This can be quantified by assessing the age of data (time since the last update) against predefined thresholds for data freshness, depending on the use case or business requirements.
Uniqueness
Uniqueness pertains to the absence of unnecessary duplicates within your data. High levels of duplicate records can indicate poor data management practices and affect the accuracy of data analysis. The duplicate record rate, calculated by identifying and counting duplicate entries as a percentage of the total data set, quantifies uniqueness.
Validity
Validity refers to how well data conforms to the specific syntax (format, type, range) defined by the data model or business rules. Validity can be quantified by checking data entries against predefined patterns or regulations and calculating the percentage of data that adheres to these criteria.
Tools and Techniques for Quantifying Data Quality
Quantifying data quality requires a blend of tools and techniques suited to the measured data quality dimensions.
- Automated Data Quality Tools: Several software solutions are designed to automate data quality measurement. These tools typically offer features for data profiling, quality scoring, anomaly detection, and cleansing. They can automatically calculate metrics for accuracy, completeness, consistency, timeliness, uniqueness, and validity based on predefined rules.
- Statistical and Machine Learning Methods: Advanced statistical analyses and machine learning models can identify patterns, anomalies, or inconsistencies in data that might not be evident through traditional methods. For example, clustering algorithms can detect duplicates or outliers, while predictive models can assess the likelihood of data being accurate based on historical trends.
- Data Profiling: Data profiling involves reviewing source data to understand its structure, content, and relationships. It helps identify issues accurately, completely, and uniquely. Organizations can generate metrics that quantify these data quality dimensions through data profiling.
Implementing a Data Quality Measurement Framework
Establishing a data quality measurement framework is essential for organizations to monitor and improve their data quality continuously. The following steps can guide this process:
- Define Data Quality Metrics: Based on the data quality dimensions relevant to the organization, define specific, measurable metrics for each dimension.
- Set Up KPIs for Data Quality: Establish Key Performance Indicators (KPIs) related to data quality aligned with business objectives. These KPIs will serve as benchmarks for assessing data quality over time.
- Regular Monitoring and Reporting: Implement a system for continuously monitoring data quality metrics and KPIs. This system should allow for the regular reporting of data quality status to stakeholders, highlighting areas of improvement and success.
Use cases
Use Case 1: Financial Services Firm Enhances Data Accuracy
A leading financial services firm faced challenges with the accuracy of its customer data, which affected loan approval processes and customer satisfaction. Within a year, the firm reduced its error rate from 5% to 0.5% by implementing a data quality measurement framework to enhance data accuracy. This improvement was quantified through regular audits and comparisons against verified data sources, leading to faster loan processing times and improved customer trust.
Use Case 2: Retail Chain Improves Inventory Management
A national retail chain needed consistency in its inventory data across multiple locations. By employing automated data quality tools to measure and improve the consistency and completeness of inventory data, the chain achieved a 95% reduction in discrepancies. This was quantified by tracking the inconsistencies monthly and implementing targeted data cleansing efforts to address the root causes.
These examples illustrate the tangible benefits of quantifying data quality across different industries, demonstrating how organizations can leverage data quality metrics to drive business improvements.
Conclusion
Quantifying data quality is not just a technical necessity; it's a strategic imperative for organizations aiming to thrive in the data-driven landscape. By understanding and applying the right metrics, tools, and frameworks, businesses can ensure their data is accurate, complete, consistent, timely, unique, and valid. While the journey to high data quality is ongoing, the benefits—from improved decision-making to enhanced customer satisfaction—are worth the effort.